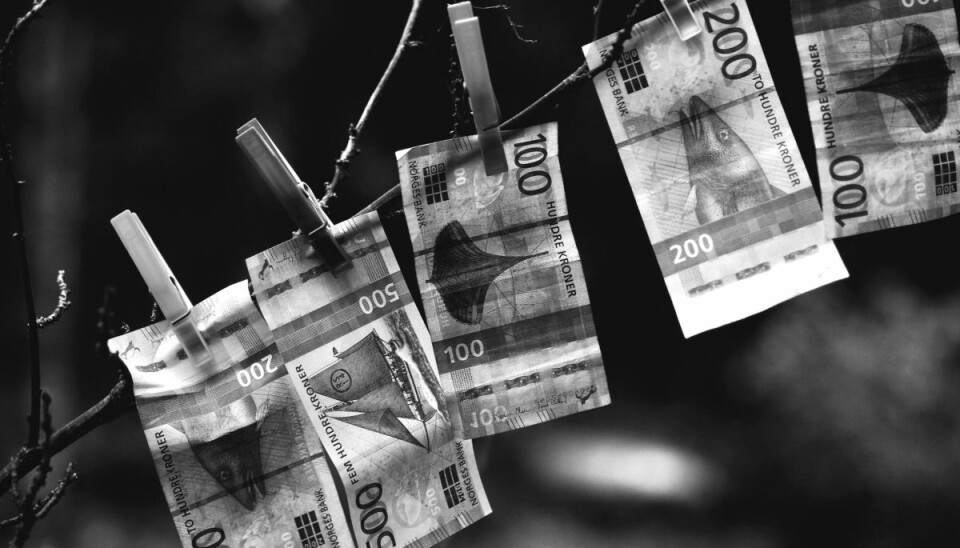
Uncertain future for fraudsters
Fraudsters who cheat on their taxes, launder money or con insurance companies are facing uncertain times. New statistical methods are increasing the likelihood that they will be caught.
This article was originally published on Apollon - Research magazine from The University of Oslo. Read the original article.
The underground economy in Norway is flourishing. Each year, at least NOK 150 billion in direct and indirect taxes is lost to evasion.
The Norwegian Tax Administration, Gjensidige Forsikring and Den norske Bank (DnB) have now joined forces with researchers at the University of Oslo and the Norwegian Computing Center to outmanoeuvre financial criminals.
Their project has been given the resounding name “Personalised Fraud Detection”.
Cheats
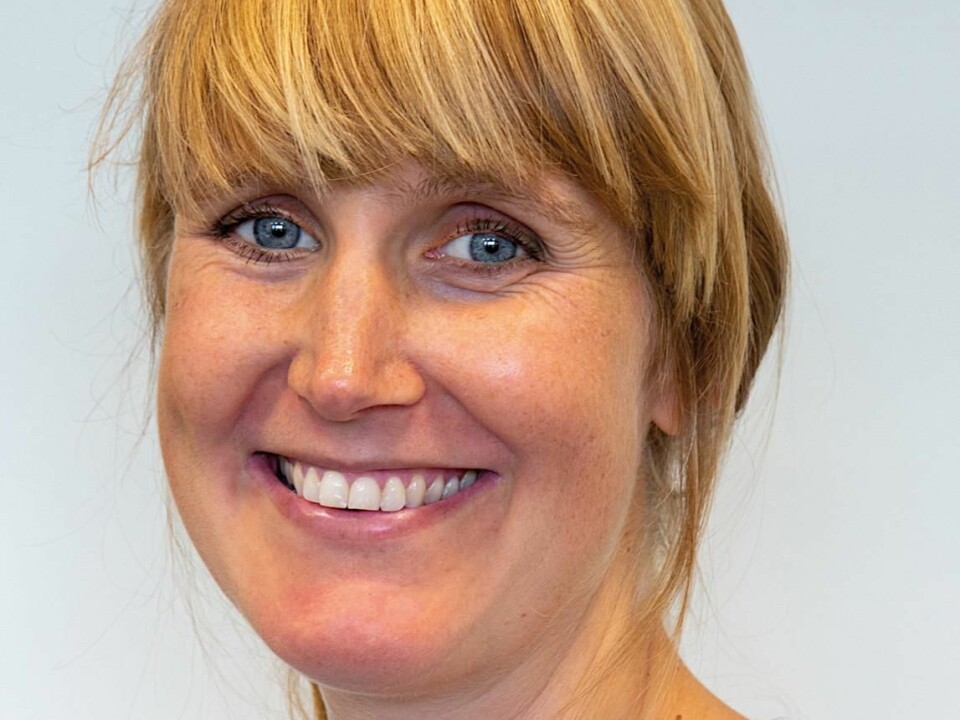
DnB needs better methods to hunt down money launderers, an activity they are legally required to report.
Gjensidige Forsikring wants to expose those who report damage that is more serious than in reality, and those who claim excessive values for stolen items.
The Tax Administration wants to adopt new methods to combat the underground economy. The project is good news for all those who do not cheat on their taxes: “Those who follow the regulations should not lose out against those who do not follow the regulations.”
Thanks to new digital capabilities, the three actors will have access to far more information than in the past. This offers tremendous opportunities.
Cheating on VAT
One of the Tax Administration’s main issues is to find new ways to expose those who cheat on reporting value-added tax (VAT).
Each year, the Tax Administration receives 1.6 million VAT returns. Many of them contain errors. The errors are caused when filers deliberately overreport expenses, underreport revenues, misunderstand the regulations or make incorrect entries.
It is impossible to manually check all VAT returns.
“The Tax Administration must therefore have an automated system to screen out prospective tax cheats. The case must then be forwarded to a case manager for more a thorough assessment,” says Ingrid Hobæk Haff, associate professor at the Department of Mathematics at the University of Oslo.
Each year, 34,000 new VAT-liable companies are established. The Tax Administration also wants to create a statistical model that can indicate the risk of who is going to cheat already from day one.
Related fraud
Although money laundering differs from insurance fraud and tax fraud, there are some similarities. The similarities will be used to develop completely new statistical methods that will uncover the swindlers.
The three collaborators are not going to exchange each other’s datasets, but by taking on different datasets with related issues, the statisticians can make strides.
Today’s methods do not work well enough. Ingrid Hobæk Haff is therefore developing new methods for exposing fraud.
“Each time a new case comes in, we want to calculate the probability of fraud,” she says.
The project is part of BigInsight, a centre for research-based innovation at UiO. The centre’s speciality is developing all-new statistical methods to uncover irregular patterns in enormous amounts of data.
The goal of the researchers is to calculate the probability that something is wrong and that the case should undergo further scrutiny.
Most attempts at fraud are never checked. Even if a case is worthy of examination, it is still not certain that it pays to check it out. The computer program will therefore simultaneously estimate the expected gain from examining the case.
Exposing the swindlers
The new statistical methods are tested on datasets whose content has already been checked. That means that the statisticians know in advance whether or not someone is a swindler.
“We can then check our results against the answer. The downside is that we don’t get to check our program on the cases that have not already been checked. The cases that were checked might have had something suspicious about them in the first place, so they weren’t selected at random,” the researcher says.
The control dataset can therefore be skewed because the cases that have been manually selected for control have already been chosen according to certain criteria.
Ingrid Hobæk Haff therefore wonders whether there is information in the rest of the dataset that complements the information they already have, and whether it is possible to exploit this information in some way.
“We must then distinguish between ‘Yes, we know the answer,’ and ‘No, we don’t know the answer’. The whole point is to find the data that stands out.”
The data that stands out doesn’t necessarily have to be fraudulent.
And although the new statistical method can expose more fraud, it is not certain they will be able to find everyone. That doesn’t matter that much.
“If we make the method just a little better, it can still lead to huge gains,” she says.
The method must also take into account that fraudsters change the way they cheat.
“If we expose fraud, the fraudsters will try other methods instead. We must therefore continually develop new methods”.
Mathematically tricky
One of the many mathematical intricacies is unbalanced datasets. Fraud cases constitute only a small percentage of all cases. Since it is difficult to pick them out, there is a risk that the case managers will be told to check out many law-abiding cases in relation to fraudulent cases.
“We only want to find the cases where the probability of fraud is high.”
Another tricky problem for the mathematicians is not only the large quantity of data, but also the many dependencies between the different data.
“Because multiple variables in a dataset can provide the same information, many of the statistical methods start to struggle.”
And as if this is not enough.
“We have a lot of information with different statistical properties. Some of the information may have all possible values and some may have only a few values. This combination can be mathematically difficult,” she tells.
Business secret
Norway is not the first country in the world to conduct research on how to detect fraud. Ingrid Hobæk Haff has therefore checked what other researchers have done.
“We have tested their methods, but they don’t solve our problems the way we would like.”
However, this does not mean that no one in the world has tried to crack the fraud code.
“I would certainly assume that big insurance companies and banks out in the big world have solved the problem with their own statistical methods. They don’t publish their solutions. They therefore remain well-guarded secrets,” says Ingrid Hobæk Haff.